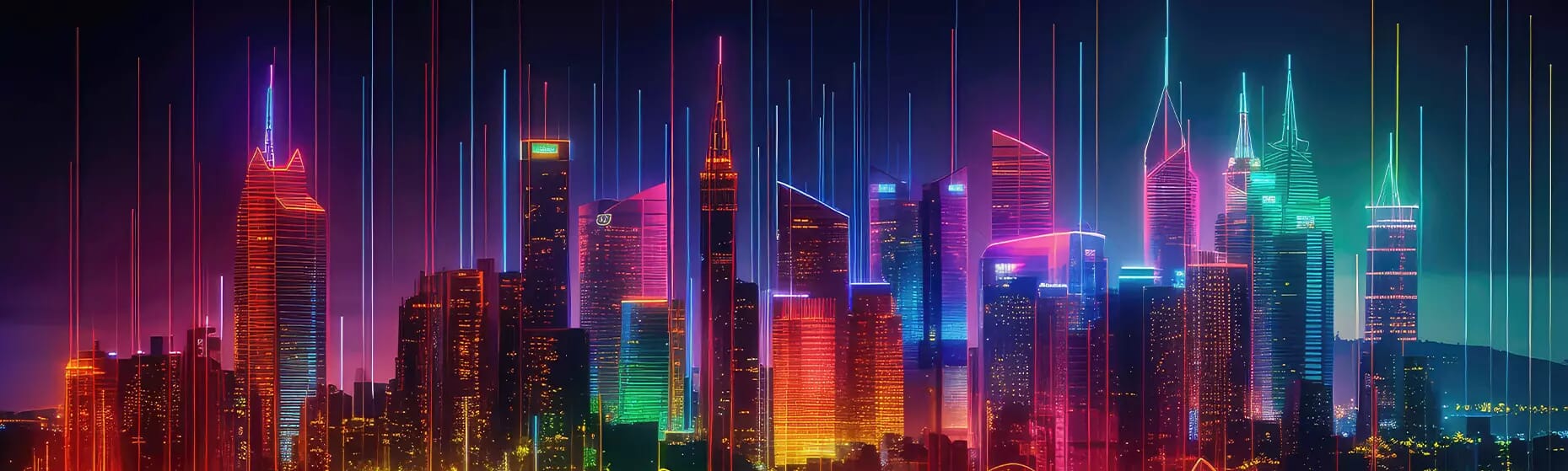
Josh Panknin on AI in Real Estate: “Stop believing the hype”
Columbia University’s Real Estate AI Research and Innovation Director shares the reality of artificial intelligence in RE
February 19, 2024Real Estate
Written by Helen Richards
Real estate has experienced a far slower adoption of AI when compared to other industries, with traditionalism in the industry having been held culprit in the past.
The reality is far more complex. It may not be the answer many real estate players want to hear, but to successfully implement AI into the real estate industry, those involved require a far deeper understanding of what AI is, what it does well and what it doesn’t do well, what it can do for the industry, and what is needed in order to implement it effectively.
Josh Panknin, Director of Real Estate AI Research and Innovation within the Engineering School at Columbia University, sat down with GRI Club ahead of co-chairing at the upcoming GRI UK & Europe Reunion 2024, to share his insights into the reality of the challenges facing real estate in the implementation of this technology.
After 15 years of experience in the real estate industry, most recently at Deutsche Bank, Josh recognised the untapped potential of using data and analytics in real estate and larger financial markets.
The Centre for AI and Business Analytics and Financial Technology at Columbia University was founded with the idea of providing a type of external R&D department to financial companies, covering not just real estate, but asset management, wealth management, and high-frequency trading.
Boasting some of the best data science and financial engineering faculty and graduate students in the world, the centre now works with JP Morgan, Deloitte, and RXR, among others, on some of the most complex real-world projects, with the potential to add real value to the real estate industry.
Read more about GRI UK & Europe Reunion 2024 here.
“These issues we’re addressing are not new to the industry, but what we’re doing will help us better understand and predict outcomes by quantifying the relationships.” (Credit: TeacherX555 | Envato)
The real estate industry isn’t adopting AI as fast as other industries, but why is that? Why is it so complex?
It's complex because real estate has constraints that are completely contradictory to what machine learning does well.
When we talk about AI, most of what’s being used today are subsets of AI called machine learning or deep learning. With those tools, we're able to take a lot of data and find patterns and relationships in that data.
You can take it a step further and you can find causal relationships. So for example, we know that markets go through cycles and that the timing of investment largely dictates returns. We know that different neighbourhoods in a larger city often grow at different rates because of gentrification. We know that we can compress cap rates slightly for properties with a highly stable cash flow and higher-credit tenants than comparable properties.
These issues we’re addressing are not new to the industry, but what we’re doing will help us better understand and predict outcomes by quantifying the relationships. Understanding cycles slightly better, identifying neighbourhoods that will experience higher rental growth rates relative to other neighbourhoods, and improving the quality of tenants and consistency of cash flow can increase yields significantly. So machine learning is really powerful when done right. This is the same approach hedge funds have used to understand the equity, debt, and derivatives markets.
The problem with machine learning is that it takes a lot of data, and real estate doesn't have a lot of data. Real estate data is broad, but not deep, meaning we have a subset of information about every building that exists, but we don’t have really deep information such as financial statements, rent rolls, the details of leases such as TI’s, early termination clauses, escalations, etc. We know this information is critical to understanding a property’s financial situation, but we don’t have access to this data outside of our particular portfolio and that’s not enough data for machine learning to use well.
An analogy would be if you were trying to make chicken parmesan for dinner and it requires 15 ingredients, but you only have 10 ingredients. You can’t make it well if you’re missing ingredients.
One reason for the lack of data is geographic constraints. The concept of similarity or “like-kind” in real estate dictates that we use properties that are close to the property we’re analysing. This shrinks the number of properties we can use as comparables pretty drastically. Another constraint is temporal. I can only use properties or information that is recent. A property that sold 5 or 10 years ago usually can’t be used as a comparable, shrinking the comparable pool even more.
We also have legal and privacy constraints like regulations and Non-Disclosure Agreements that limit the amount of information that can be shared publicly.
Then we have qualitative data, things like the view. So in New York, if you're in a high rise on the 40th floor, you have a much better view than somebody on the 10th floor. And we know that that view is a really big input. It's a really big factor in the ultimate value of that apartment, but it’s very difficult for us to quantify the value of that view at scale.
When you use machine learning you have to be explicitly clear. You have to be very detailed about what you're asking for. Despite the popular narrative, AI is not magic. It’s mathematics. AI and machine learning are incredible at finding patterns and relationships in data, but it doesn’t magically create data and it doesn’t work well when relevant factors are missing from the data it is provided.
The environment that we have in real estate in terms of data constraints and the types of data - including being in a visual and spatial world - makes it really difficult for AI and machine learning to work well for many use cases.
Another reason we haven’t seen a big impact from technology in real estate is because as you get into more complex parts of the real estate world you need more complex technology. We have a lot of simplistic functions and simplistic applications that are good for what they do, but when you try to move up into more complex things, most of these applications start to fail.
When you have real estate people who don't understand technology and you have technology people who don't understand real estate, it becomes very difficult to build really sophisticated and nuanced applications.
A lot of these companies will pilot these technologies and startups, and they'll say it's just not good enough; it's not flexible enough; it doesn't capture all the things we want to capture. So it doesn't get implemented within the company.
If it's a problem with data and a lack of expertise on both sides, how do we fix that? Is AI in real estate progressing?
I have a bit of a contrarian view. There's a quote I really like from the book “The Techno-Human Condition”: “We are well aware that the standard approach is to discover deep problems and issues, then offer vague, tentative, or often utterly impractical solutions that pale next to the challenge identified by the analysis…”.
We can apply this to real estate problems: climate change, ESG, GenAI - every year you have a new shiny object that pops out. Every year you have some new trend that's out there that people are throwing money at.
On one side you have startups that are taking advantage of that, and they're raising money on the back of whatever shiny object is there. But these things take a lot of work to solve; these are big problems; they're worthy problems; we should be addressing them.
But you're likely not going to solve them with a startup with an app. These problems require a lot of funding, deep research on new materials, new technologies, new implementations, new infrastructure to develop. And that's not happening.
Most of the $120 billion that's been put out (depending on the source), has been into venture capital or investment in startups. I don’t think you’re going to solve major problems by investing in a startup with an app that has a seven-year horizon, looking for a 10X return.
I think one of the reasons that's the case is that real estate people don't have technical backgrounds and technical people don't have real estate backgrounds. So, if I'm on the technology side and I come up to somebody in the real estate side and say ‘I have this idea to implement and it's going to fix your problem’, real estate people don't have the ability to vet that proposal or that solution. They don't have the ability to say ‘I don't think that's going to work based on whatever factors’.
The proptech world has been very good at creating FOMO and hype and saying you've got to invest now or you're going to miss out. Your competitors are piloting this thing.
In my conversations with real estate companies, I haven’t heard of much progress being made. Everybody's looking for something transformative, but I haven’t heard of anyone finding that. Everybody thinks that their competitor has it figured out. And those competitors think that everybody else has it figured out, but it seems that in reality everyone is struggling to create real impact and real value with technology.
We need to step back and take a much more sophisticated approach. In our opinion, that starts with the educational side. People on the real estate side need to understand more about what they're investing in before they throw tens or hundreds of millions or billions of dollars at ideas without vetting the feasibility of those ideas. Many of the companies that have been started were never technically feasible, so the money/time/resources invested could have been saved or put into something more viable.
Is the research centre at Columbia University the first of its kind or are there other research centres focusing on the same research?
It's the only one that I know of that's being run out of an engineering school, and we are different in what we do. There are a lot of real estate programmes in the world, but they're more traditional real estate and finance focused. Graduates go to Wall Street and hedge funds coming out of them.
We're different in the sense that we're taking a very technical approach to solving these problems and understanding these problems. Our students are all engineers, they all come in with bachelor's degrees in applied mathematics, physics, computer science, econometrics, statistics, and they’re great coders/programmers.
They're very technical and quantitative when they come in, and we are trying to teach them the real estate side. How do you work with real estate data? What are some of the problems so that they can use that background that they have more effectively in the real estate industry?
The students come out of project-based classes, so they attend lectures on AI, machine learning, deep learning in quantitative finance, etc. and then apply those lessons to real-world projects building AI, machine learning, and deep learning applications.
They're able to go into companies on day one and start making an impact because they not only have a strong data science background, but they have enough real estate background from our projects to be able to integrate within these real estate companies and understand what's going on.
When they get to that company, they know very well what constraints they have, what restrictions they have, and what they need to do to work around those constraints and challenges in order to be successful, but also to be able to give feedback to these companies about what will work and what won’t.
Many of the things that these companies are asking for are just not doable. You can't use machine learning if you don't have data. These students are better able to look at a problem and say ‘we don't have enough data for this’, and while that company may not get the outcome they wanted, at least they didn't waste their time and money trying to develop something that was going to fail from the beginning. We’re trying to produce students who have the ability to translate better between the real estate side and the technical side.
What would your advice to the real estate industry be? How should AI be pursued in real estate?
That’s a very difficult question because in order for most people to ingest the advice that I have, they need to be shown this stuff, not told this stuff, because they’re being told two different things.
On one side, on the PropTech side, they’re being told that everything is transformative, revolutionary, and disruptive - and if they don’t jump on it, they’re going to be behind. Then on the other side, we’re saying ‘no, that doesn’t work that way; you’re not getting the kinds of results that you’re looking for with that approach’.
If I had to synthesise it I would say stop believing the hype and the FOMO; it’s not working. We’ve had almost 15 years now of the same narrative and it is not working. It is not the right approach to developing sophisticated technology.
The other is to pursue education and knowledge on what you’re investing in, because in my opinion, 90% of the money that has been put out has been put out to projects that failed before they even started for a variety of reasons.
There's a lot of value to be had in use cases that are more mundane - automation, efficiency, basic analytics - but everybody wants to chase the magical flying rainbow unicorn that's going to result in a 10X return.
Most of those things are going to fail. Take a more mundane approach to build a strong foundation of data and analytics and five years down the road your company will look vastly different than it does now.
There are many use cases of automation that are providing a lot of efficiency. But you don't hear about them because they're not exciting; they don’t sound transformative. But that is the way companies are really going to transform, by building those tools internally and building foundations rather than trying to find the next hot startup.
Companies don’t understand that it’s not likely they’ll develop a competitive advantage by adopting external technologies. Startups and third-parties who develop good technology are trying to sell those products and services to as many customers as possible, including your competitors.
For example, if you’re a multifamily investment company and a startup develops really good acquisitions software, they’re trying to sell that software to you and to every other company who acquires multifamily properties. So by adopting that software, the best you can do is to maintain the status quo with your competitors. Competitive advantage comes from developing tools internally that are core to your long-term strategy as a firm.
Are there ethical considerations to be addressed?
We still know so little about many AI, machine learning, deep learning outcomes - and what they do - that it's hard to even recognize what some of those ethical considerations are. Dr. Ali Hirsa, the Director of our Research Centre, is very big on “explainable AI” or “interpretable AI,” meaning figuring out why we got the output we did from AI. We don’t just get an output and stop there, but we use it to ask better questions about what we’re analysing, what the implications are, and how we can use the output to better understand the concept.
We find patterns in data. Let's go back and ask questions about what we're getting, not just say, here's some data, that's the answer and call it a day. I think that is a really good approach for us to be able to understand what it is that we're doing. And I think that approach would be valuable for the ethical side of it. What are we doing? What are the implications?
How about in terms of regulation?
Should we stop progress? Those are much larger questions than I'm capable of answering, but we think about it often. What would be the effect of the thing that we developed? How could we use it? What is going to be useful for? Who’s going to use it? How is that going to change companies? Those are all questions that we ask, and hopefully we come up with the right ones.
Josh Panknin will be co-chairing the AI, Tech & Data panel at the upcoming GRI UK & Europe Reunion 2024 on February 21-22 at Four Seasons London.
Read more and register to join the discussion.
Real estate has experienced a far slower adoption of AI when compared to other industries, with traditionalism in the industry having been held culprit in the past.
The reality is far more complex. It may not be the answer many real estate players want to hear, but to successfully implement AI into the real estate industry, those involved require a far deeper understanding of what AI is, what it does well and what it doesn’t do well, what it can do for the industry, and what is needed in order to implement it effectively.
Josh Panknin, Director of Real Estate AI Research and Innovation within the Engineering School at Columbia University, sat down with GRI Club ahead of co-chairing at the upcoming GRI UK & Europe Reunion 2024, to share his insights into the reality of the challenges facing real estate in the implementation of this technology.
After 15 years of experience in the real estate industry, most recently at Deutsche Bank, Josh recognised the untapped potential of using data and analytics in real estate and larger financial markets.
The Centre for AI and Business Analytics and Financial Technology at Columbia University was founded with the idea of providing a type of external R&D department to financial companies, covering not just real estate, but asset management, wealth management, and high-frequency trading.
Boasting some of the best data science and financial engineering faculty and graduate students in the world, the centre now works with JP Morgan, Deloitte, and RXR, among others, on some of the most complex real-world projects, with the potential to add real value to the real estate industry.
Read more about GRI UK & Europe Reunion 2024 here.
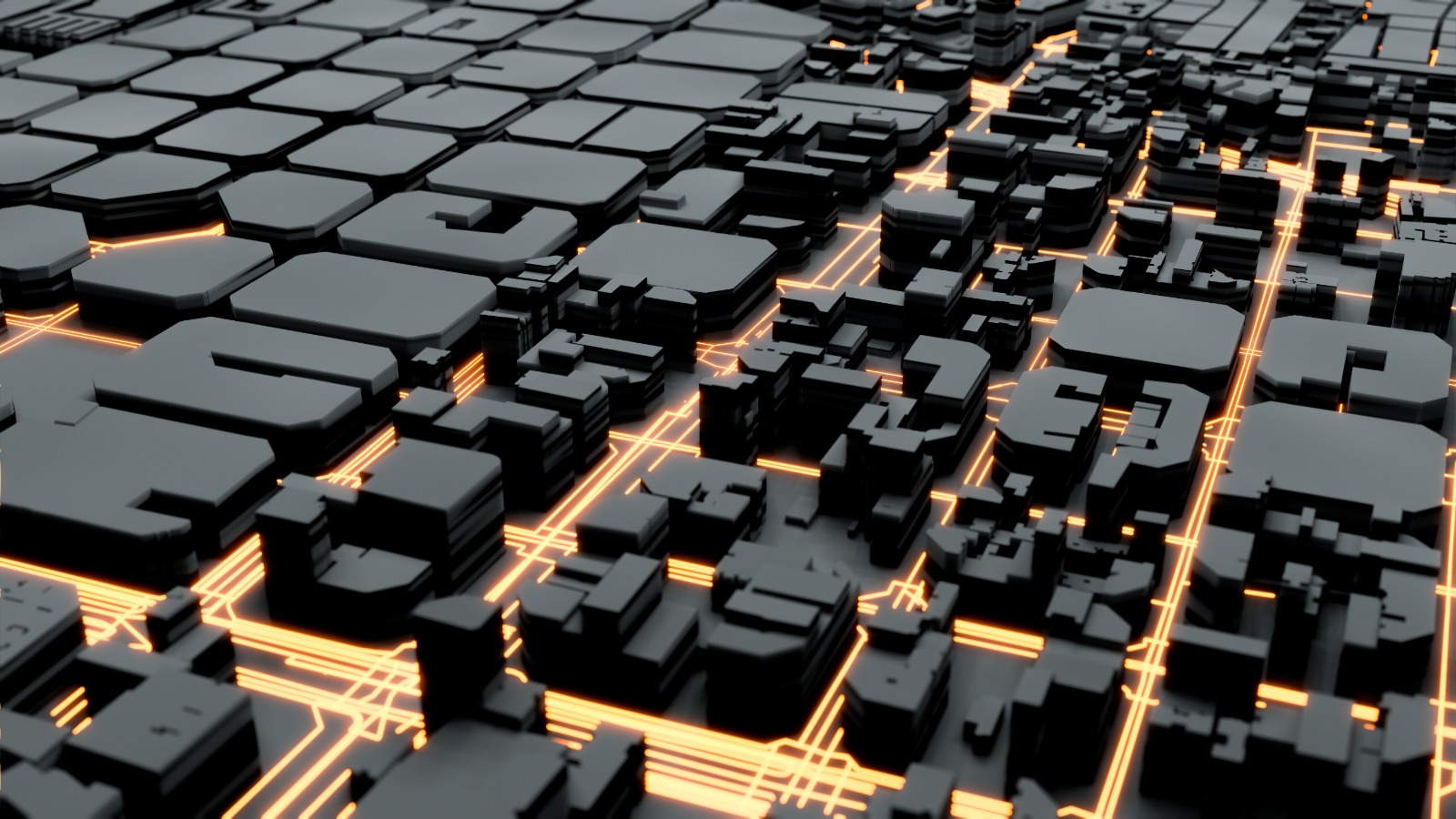
The real estate industry isn’t adopting AI as fast as other industries, but why is that? Why is it so complex?
It's complex because real estate has constraints that are completely contradictory to what machine learning does well.
When we talk about AI, most of what’s being used today are subsets of AI called machine learning or deep learning. With those tools, we're able to take a lot of data and find patterns and relationships in that data.
You can take it a step further and you can find causal relationships. So for example, we know that markets go through cycles and that the timing of investment largely dictates returns. We know that different neighbourhoods in a larger city often grow at different rates because of gentrification. We know that we can compress cap rates slightly for properties with a highly stable cash flow and higher-credit tenants than comparable properties.
These issues we’re addressing are not new to the industry, but what we’re doing will help us better understand and predict outcomes by quantifying the relationships. Understanding cycles slightly better, identifying neighbourhoods that will experience higher rental growth rates relative to other neighbourhoods, and improving the quality of tenants and consistency of cash flow can increase yields significantly. So machine learning is really powerful when done right. This is the same approach hedge funds have used to understand the equity, debt, and derivatives markets.
The problem with machine learning is that it takes a lot of data, and real estate doesn't have a lot of data. Real estate data is broad, but not deep, meaning we have a subset of information about every building that exists, but we don’t have really deep information such as financial statements, rent rolls, the details of leases such as TI’s, early termination clauses, escalations, etc. We know this information is critical to understanding a property’s financial situation, but we don’t have access to this data outside of our particular portfolio and that’s not enough data for machine learning to use well.
An analogy would be if you were trying to make chicken parmesan for dinner and it requires 15 ingredients, but you only have 10 ingredients. You can’t make it well if you’re missing ingredients.
One reason for the lack of data is geographic constraints. The concept of similarity or “like-kind” in real estate dictates that we use properties that are close to the property we’re analysing. This shrinks the number of properties we can use as comparables pretty drastically. Another constraint is temporal. I can only use properties or information that is recent. A property that sold 5 or 10 years ago usually can’t be used as a comparable, shrinking the comparable pool even more.
We also have legal and privacy constraints like regulations and Non-Disclosure Agreements that limit the amount of information that can be shared publicly.
Then we have qualitative data, things like the view. So in New York, if you're in a high rise on the 40th floor, you have a much better view than somebody on the 10th floor. And we know that that view is a really big input. It's a really big factor in the ultimate value of that apartment, but it’s very difficult for us to quantify the value of that view at scale.
When you use machine learning you have to be explicitly clear. You have to be very detailed about what you're asking for. Despite the popular narrative, AI is not magic. It’s mathematics. AI and machine learning are incredible at finding patterns and relationships in data, but it doesn’t magically create data and it doesn’t work well when relevant factors are missing from the data it is provided.
The environment that we have in real estate in terms of data constraints and the types of data - including being in a visual and spatial world - makes it really difficult for AI and machine learning to work well for many use cases.
Another reason we haven’t seen a big impact from technology in real estate is because as you get into more complex parts of the real estate world you need more complex technology. We have a lot of simplistic functions and simplistic applications that are good for what they do, but when you try to move up into more complex things, most of these applications start to fail.
When you have real estate people who don't understand technology and you have technology people who don't understand real estate, it becomes very difficult to build really sophisticated and nuanced applications.
A lot of these companies will pilot these technologies and startups, and they'll say it's just not good enough; it's not flexible enough; it doesn't capture all the things we want to capture. So it doesn't get implemented within the company.
If it's a problem with data and a lack of expertise on both sides, how do we fix that? Is AI in real estate progressing?
I have a bit of a contrarian view. There's a quote I really like from the book “The Techno-Human Condition”: “We are well aware that the standard approach is to discover deep problems and issues, then offer vague, tentative, or often utterly impractical solutions that pale next to the challenge identified by the analysis…”.
We can apply this to real estate problems: climate change, ESG, GenAI - every year you have a new shiny object that pops out. Every year you have some new trend that's out there that people are throwing money at.
On one side you have startups that are taking advantage of that, and they're raising money on the back of whatever shiny object is there. But these things take a lot of work to solve; these are big problems; they're worthy problems; we should be addressing them.
But you're likely not going to solve them with a startup with an app. These problems require a lot of funding, deep research on new materials, new technologies, new implementations, new infrastructure to develop. And that's not happening.
Most of the $120 billion that's been put out (depending on the source), has been into venture capital or investment in startups. I don’t think you’re going to solve major problems by investing in a startup with an app that has a seven-year horizon, looking for a 10X return.
I think one of the reasons that's the case is that real estate people don't have technical backgrounds and technical people don't have real estate backgrounds. So, if I'm on the technology side and I come up to somebody in the real estate side and say ‘I have this idea to implement and it's going to fix your problem’, real estate people don't have the ability to vet that proposal or that solution. They don't have the ability to say ‘I don't think that's going to work based on whatever factors’.
The proptech world has been very good at creating FOMO and hype and saying you've got to invest now or you're going to miss out. Your competitors are piloting this thing.
In my conversations with real estate companies, I haven’t heard of much progress being made. Everybody's looking for something transformative, but I haven’t heard of anyone finding that. Everybody thinks that their competitor has it figured out. And those competitors think that everybody else has it figured out, but it seems that in reality everyone is struggling to create real impact and real value with technology.
We need to step back and take a much more sophisticated approach. In our opinion, that starts with the educational side. People on the real estate side need to understand more about what they're investing in before they throw tens or hundreds of millions or billions of dollars at ideas without vetting the feasibility of those ideas. Many of the companies that have been started were never technically feasible, so the money/time/resources invested could have been saved or put into something more viable.
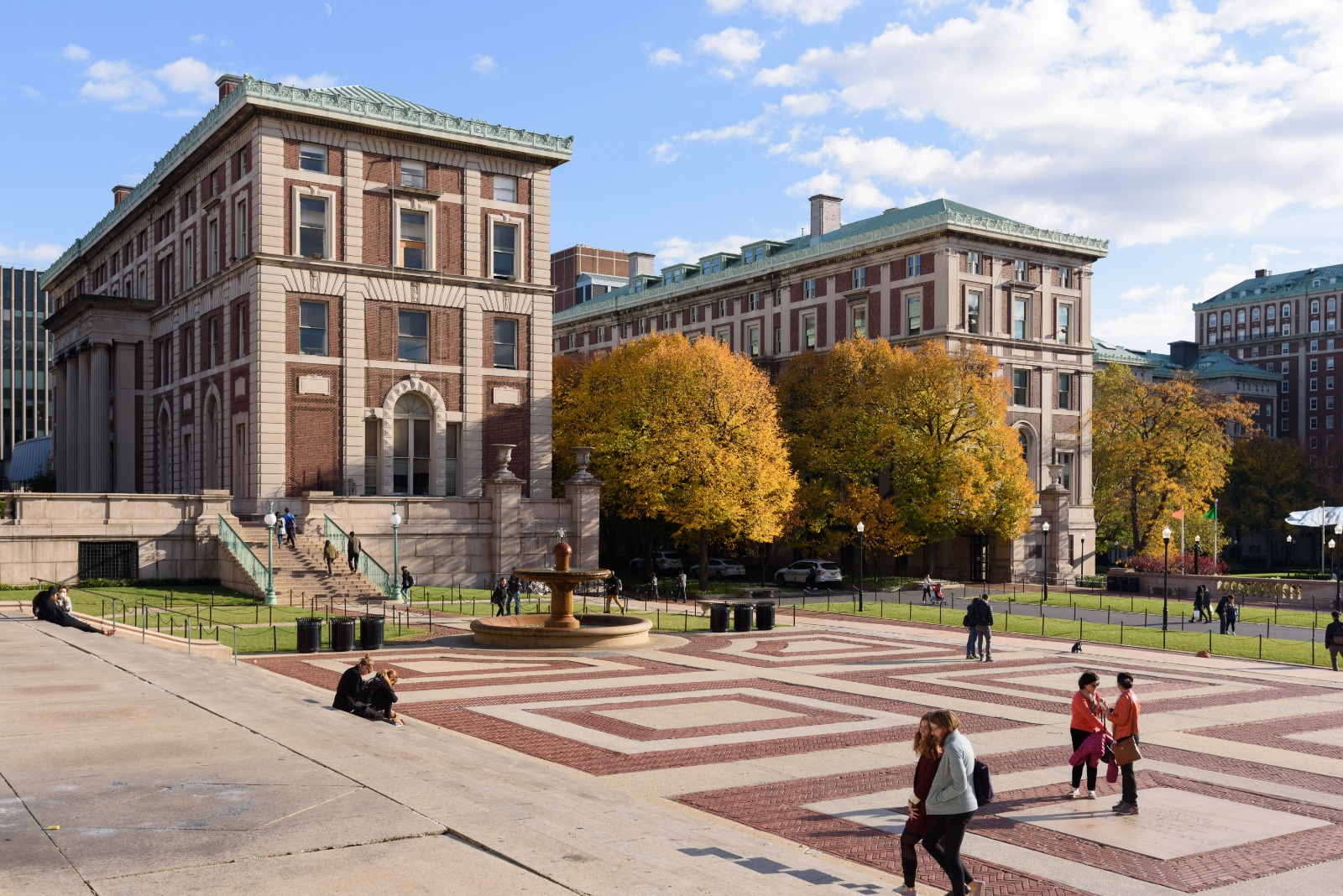
The Centre for AI and Business Analytics and Financial Technology is based out of Columbia University, New York (Credit: King of Hearts | WikiCommons)
Is the research centre at Columbia University the first of its kind or are there other research centres focusing on the same research?
It's the only one that I know of that's being run out of an engineering school, and we are different in what we do. There are a lot of real estate programmes in the world, but they're more traditional real estate and finance focused. Graduates go to Wall Street and hedge funds coming out of them.
We're different in the sense that we're taking a very technical approach to solving these problems and understanding these problems. Our students are all engineers, they all come in with bachelor's degrees in applied mathematics, physics, computer science, econometrics, statistics, and they’re great coders/programmers.
They're very technical and quantitative when they come in, and we are trying to teach them the real estate side. How do you work with real estate data? What are some of the problems so that they can use that background that they have more effectively in the real estate industry?
The students come out of project-based classes, so they attend lectures on AI, machine learning, deep learning in quantitative finance, etc. and then apply those lessons to real-world projects building AI, machine learning, and deep learning applications.
They're able to go into companies on day one and start making an impact because they not only have a strong data science background, but they have enough real estate background from our projects to be able to integrate within these real estate companies and understand what's going on.
When they get to that company, they know very well what constraints they have, what restrictions they have, and what they need to do to work around those constraints and challenges in order to be successful, but also to be able to give feedback to these companies about what will work and what won’t.
Many of the things that these companies are asking for are just not doable. You can't use machine learning if you don't have data. These students are better able to look at a problem and say ‘we don't have enough data for this’, and while that company may not get the outcome they wanted, at least they didn't waste their time and money trying to develop something that was going to fail from the beginning. We’re trying to produce students who have the ability to translate better between the real estate side and the technical side.
What would your advice to the real estate industry be? How should AI be pursued in real estate?
That’s a very difficult question because in order for most people to ingest the advice that I have, they need to be shown this stuff, not told this stuff, because they’re being told two different things.
On one side, on the PropTech side, they’re being told that everything is transformative, revolutionary, and disruptive - and if they don’t jump on it, they’re going to be behind. Then on the other side, we’re saying ‘no, that doesn’t work that way; you’re not getting the kinds of results that you’re looking for with that approach’.
If I had to synthesise it I would say stop believing the hype and the FOMO; it’s not working. We’ve had almost 15 years now of the same narrative and it is not working. It is not the right approach to developing sophisticated technology.
The other is to pursue education and knowledge on what you’re investing in, because in my opinion, 90% of the money that has been put out has been put out to projects that failed before they even started for a variety of reasons.
There's a lot of value to be had in use cases that are more mundane - automation, efficiency, basic analytics - but everybody wants to chase the magical flying rainbow unicorn that's going to result in a 10X return.
Most of those things are going to fail. Take a more mundane approach to build a strong foundation of data and analytics and five years down the road your company will look vastly different than it does now.
There are many use cases of automation that are providing a lot of efficiency. But you don't hear about them because they're not exciting; they don’t sound transformative. But that is the way companies are really going to transform, by building those tools internally and building foundations rather than trying to find the next hot startup.
Companies don’t understand that it’s not likely they’ll develop a competitive advantage by adopting external technologies. Startups and third-parties who develop good technology are trying to sell those products and services to as many customers as possible, including your competitors.
For example, if you’re a multifamily investment company and a startup develops really good acquisitions software, they’re trying to sell that software to you and to every other company who acquires multifamily properties. So by adopting that software, the best you can do is to maintain the status quo with your competitors. Competitive advantage comes from developing tools internally that are core to your long-term strategy as a firm.
Are there ethical considerations to be addressed?
We still know so little about many AI, machine learning, deep learning outcomes - and what they do - that it's hard to even recognize what some of those ethical considerations are. Dr. Ali Hirsa, the Director of our Research Centre, is very big on “explainable AI” or “interpretable AI,” meaning figuring out why we got the output we did from AI. We don’t just get an output and stop there, but we use it to ask better questions about what we’re analysing, what the implications are, and how we can use the output to better understand the concept.
We find patterns in data. Let's go back and ask questions about what we're getting, not just say, here's some data, that's the answer and call it a day. I think that is a really good approach for us to be able to understand what it is that we're doing. And I think that approach would be valuable for the ethical side of it. What are we doing? What are the implications?
How about in terms of regulation?
Should we stop progress? Those are much larger questions than I'm capable of answering, but we think about it often. What would be the effect of the thing that we developed? How could we use it? What is going to be useful for? Who’s going to use it? How is that going to change companies? Those are all questions that we ask, and hopefully we come up with the right ones.
Josh Panknin will be co-chairing the AI, Tech & Data panel at the upcoming GRI UK & Europe Reunion 2024 on February 21-22 at Four Seasons London.
Read more and register to join the discussion.